Announcing Krush 1.0
Announcing Krush – idiomatic persistence layer for Kotlin, based on Exposed
How we use Kotlin with Exposed at TouK
Analyzing 2018 World Cup match data with Clojure
When distributed monolith may work for you
For sure following hype just for being “hot” can bring problems.
Sputnik lands on GitHub!
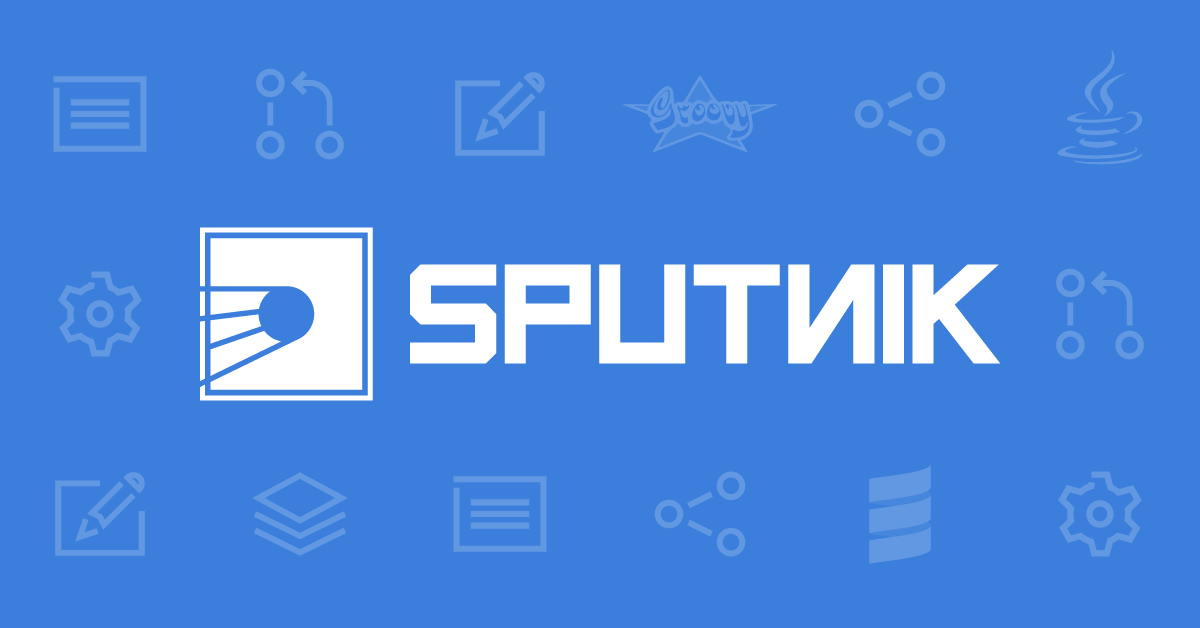
Clojure web development – state of the art – part 2
Clojure web development – state of the art
It’s now more than a year that I’m getting familiar with Clojure and the more I dive into it, the more it becomes the language. Once you defeat the “parentheses fear”, everything else just makes the difference: tooling, community, good engineering practices. So it’s now time for me to convince others. In this post I’ll try to walktrough a simple web application from scratch to show key tools and libraries used to develop with Clojure in late 2015.
Note for Clojurians: This material is rather elementary and may be useful for you if you already know Clojure a bit but never did anything bigger than hello world application.
Note for Java developers: This material shows how to replace Spring, Angular, grunt, live-reload with a bunch of Clojure tools and libraries and a bit of code.
The repo with final code and individual steps is here.
Bootstrap
I think all agreed that component is the industry standard for managing lifecycle of Clojure applications. If you are a Java developer you may think of it as a Spring (DI) replacement - you declare dependencies between “components” which are resolved on “system” startup. So you just say “my component needs a repository/database pool” and component library “injects” it for you.
To keep things simple I like to start with duct web app template. It’s a nice starter component application following the 12-factor philosophy. So let’s start with it:
lein new duct clojure-web-app +example
The +example
parameter tells duct to create an example endpoint with HTTP routes - this would be helpful. To finish bootstraping run lein setup
inside clojure-web-app
directory.
Ok, let’s dive into the code. Component and injection related code should be in system.clj
file:
(defn new-system [config]
(let [config (meta-merge base-config config)]
(-> (component/system-map
:app (handler-component (:app config))
:http (jetty-server (:http config))
:example (endpoint-component example-endpoint))
(component/system-using
{:http [:app]
:app [:example]
:example []}))))
In the first section you instantiate components without dependencies, which are resolved in the second section. So in this example, “http” component (server) requires “app” (application abstraction), which in turn is injected with “example” (actual routes). If your component needs others, you just can get then by names (precisely: by Clojure keywords).
To start the system you must fire a REPL - interactive environment running within context of your application:
lein repl
After seeing prompt type (go)
. Application should start, you can visit http://localhost:3000 to see some example page.
A huge benefit of using component approach is that you get fully reloadable application. When you change literally anything - configuration, endpoints, implementation, you can just type (reset)
in REPL and your application is up-to-date with the code. It’s a feature of the language, no JRebel, Spring-reloaded needed.
Adding REST endpoint
Ok, in the next step let’s add some basic REST endpoint returning JSON. We need to add 2 dependencies in project.clj
file:
:dependencies
...
[ring/ring-json "0.3.1"]
[cheshire "5.1.1"]
Ring-json adds support for JSON for your routes (in ring it’s called middleware) and cheshire is Clojure JSON parser (like Jackson in Java). Modifying project dependencies if one of the few tasks that require restarting the REPL, so hit CTRL-C and type lein repl
again.
To configure JSON middleware we have to add wrap-json-body
and wrap-json-response
just before wrap-defaults
in system.clj
:
(:require
...
[ring.middleware.json :refer [wrap-json-body wrap-json-response]])
(def base-config
{:app {:middleware [[wrap-not-found :not-found]
[wrap-json-body {:keywords? true}]
[wrap-json-response]
[wrap-defaults :defaults]]
And finally, in endpoint/example.clj
we must add some route with JSON response:
(:require
...
[ring.util.response :refer [response]]))
(defn example-endpoint [config]
(routes
(GET "/hello" [] (response {:hello "world"}))
...
Reload app with (reset)
in REPL and test new route with curl
:
curl -v http://localhost:3000/hello
< HTTP/1.1 200 OK
< Date: Tue, 15 Sep 2015 21:17:37 GMT
< Content-Type: application/json; charset=utf-8
< Set-Cookie: ring-session=37c337fb-6bbc-4e65-a060-1997718d03e0;Path=/;HttpOnly
< X-XSS-Protection: 1; mode=block
< X-Frame-Options: SAMEORIGIN
< X-Content-Type-Options: nosniff
< Content-Length: 151
* Server Jetty(9.2.10.v20150310) is not blacklisted
< Server: Jetty(9.2.10.v20150310)
<
* Connection #0 to host localhost left intact
{"hello": "world"}
It works! In case of any problems you can find working version in this commit.
Adding frontend with figwheel
Coding backend in Clojure is great, but what about the frontend? As you may already know, Clojure could be compiled not only to JVM bytecode, but also to Javascript. This may sound familiar if you used e.g. Coffescript. But ClojureScript philosophy is not only to provide some syntax sugar, but improve your development cycle with great tooling and fully interactive development. Let’s see how to achieve it.
The best way to introduce ClojureScript to a project is figweel. First let’s add fighweel plugin and configuration to project.clj
:
:plugins
...
[lein-figwheel "0.3.9"]
And cljsbuild configuration:
:cljsbuild
{:builds [{:id "dev"
:source-paths ["src-cljs"]
:figwheel true
:compiler {:main "clojure-web-app.core"
:asset-path "js/out"
:output-to "resources/public/js/clojure-web-app.js"
:output-dir "resources/public/js/out"}}]}
In short this tells ClojureScript compiler to take sources from src-cljs
with figweel
support and but resulting JavaScript into resources/public/js/clojure-web-app.js
file. So we need to include this file in a simple HTML page:
<!DOCTYPE html>
<head>
</head>
<body>
<div id="main">
</div>
<script src="js/clojure-web-app.js" type="text/javascript"></script>
</body>
</html>
To serve this static file we need to change some defaults and add corresponding route. In system.clj
change api-defaults
to site-defaults
both in require section and base-config
function. In example.clj
add following route:
(GET "/" [] (io/resource "public/index.html")
Again (reset)
in REPL window should reload everything.
But where is our ClojureScript source file? Let’s create file core.cljs
in src-cljs/clojure-web-app
directory:
(ns ^:figwheel-always clojure-web-app.core)
(enable-console-print!)
(println "hello from clojurescript")
Open another terminal and run lein fighweel
. It should compile ClojureScript and print ‘Prompt will show when figwheel connects to your application’. Open http://localhost:3000
. Fighweel window should prompt:
To quit, type: :cljs/quit
cljs.user=>
Type (js/alert "hello")
. Boom! If everything worked you should see and alert in your browser. Open developers console in your browser. You should see hello from clojurescript
printed on the console. Change it in core.cljs
to (println "fighweel rocks")
and save the file. Without reloading the page your should see updated message. Figweel rocks! Again, in case of any problems, refer to this commit.
In the next post I’ll show how to fetch data from MongoDB, serve it with REST to the broser and write ReactJs/Om components to render it. Stay tuned! Read more